Self-driving product understanding for thousands of categories
Knowledge graphs have been used to support a wide range of applications and enhance search results for multiple major search engines, such as Google and Bing. At Amazon we are building a Product Graph, an authoritative knowledge graph for all products in the world. The thousands of product verticals we need to model, the vast number of data sources we need to extract knowledge from, the huge volume of new products we need to handle every day, and the various applications in Search, Discovery, Personalization, Voice, that we wish to support, all present big challenges in constructing such a graph. In this talk we describe our efforts for self-driving knowledge collection for products of thousands of types. The system includes a suite of novel techniques for taxonomy construction, product property identification, knowledge extraction, anomaly detection, and synonym discovery. Our system is a) automatic, requiring little human intervention, b) multi-scalable, scalable in multiple dimensions including many domains, products, and attributes, and c) integrative, exploiting rich customer behavior logs. We describe what we learned in building this product graph and applying it to support customer-facing applications.
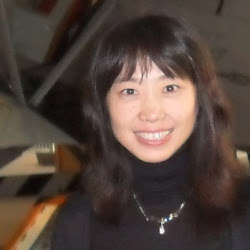
Xin Luna Dong
Xin Luna Dong is a Senior Principal Scientist at Amazon, leading the efforts of constructing Amazon Product Knowledge Graph. She was one of the major contributors to the Google Knowledge Vault project, and has led the Knowledge-based Trust project, which is called the “Google Truth Machine” by Washington’s Post. She has co-authored book “Big Data Integration”, was awarded ACM Distinguished Member, and VLDB Early Career Research Contribution Award for “advancing the state of the art of knowledge fusion”. She serves in VLDB endowment and PVLDB advisory committee, and is a PC co-chair for WSDM'2022, VLDB'2021, KDD'2020 ADS Invited Talk Series.