Toward Activity Discovery in the Personal Web
Individuals' personal information collections (their emails, files, appointments, web searches, contacts, etc) offer a wealth of insights into the organization and structure of their everyday lives. In this talk, I address the task of learning representations of personal information items to capture individuals' ongoing activities---i.e., projects and tasks. Such representations can be used in "activity-centric" applications like personal assistants, email clients, and productivity tools to help people better manage their data and time. I will outline our graph-based modeling approach, which leverages the inherent interconnected structure of personal information collections, and show that efficient, exact techniques can be derived to incrementally update representations as new data arrive. Finally, I will demonstrate the strengths of our approach against competitive baselines in both a novel intrinsic rating task and an extrinsic personal recommendation task.
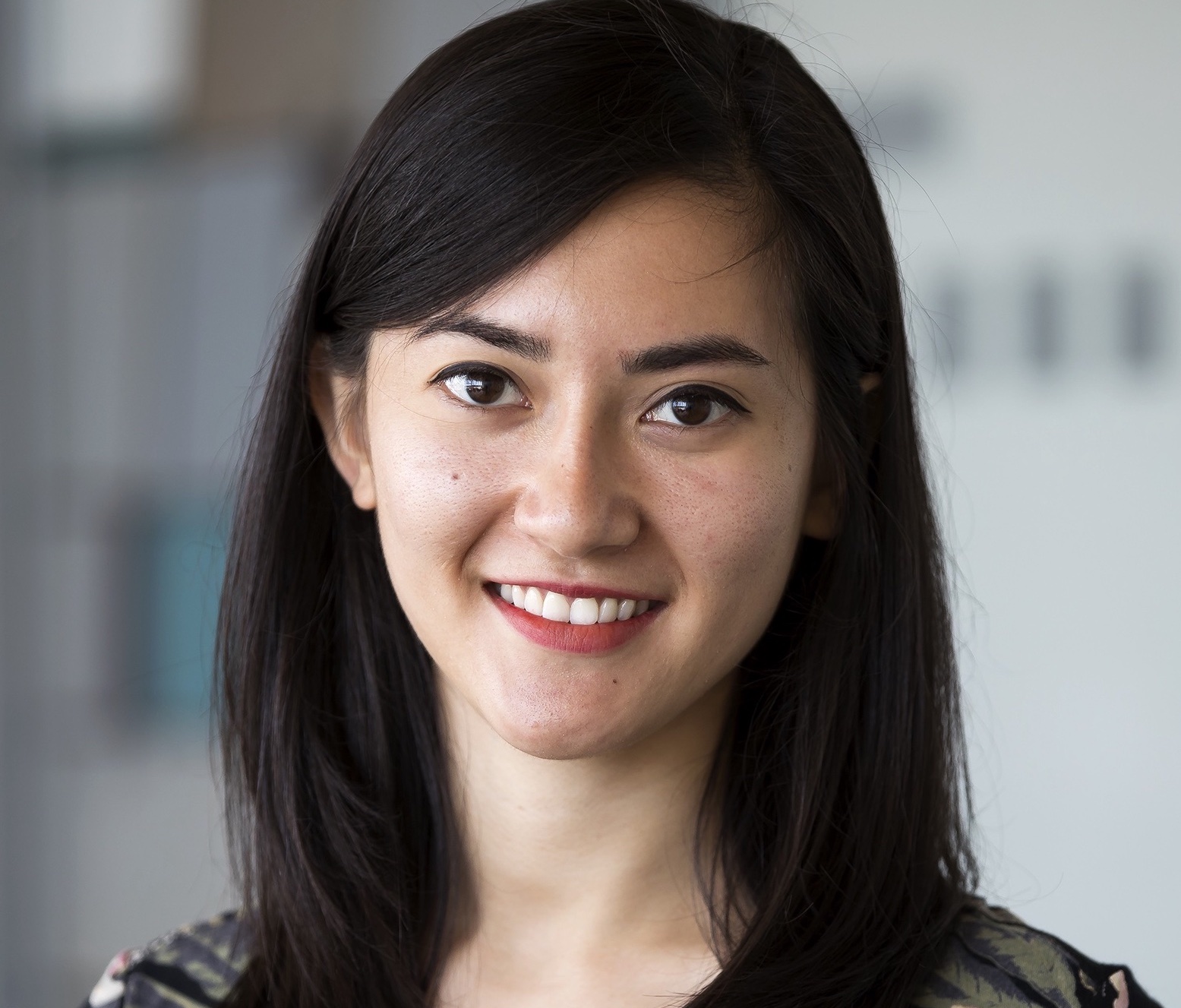
Tara Safavi
Tara is a PhD candidate in computer science at the University of Michigan working with Danai Koutra. Her research, which is at the intersection of graph-based machine learning and natural language processing, focuses on relational knowledge representation and reasoning in machines. She is currently supported by a National Science Foundation Graduate Research Fellowship and a Google Women Techmakers scholarship, and received a Best Student Paper award at the IEEE ICDM conference in 2019.